As an academic support unit, we are in the business of helping others. But it goes beyond simply service – we help instructors to help themselves. The reach and scope of our services can feel quite large since teaching and learning are so foundational to the university, and we receive numerous requests for our assistance. Our staff members’ interests and ideas for projects are also quite broad. However, sometimes we have to say no to requests we receive or ideas we generate. Is this ever a good idea? Continue reading The Value of Saying No: An Exercise in Reframing — Donna Ellis
Author: Mark Morton
CTE’s 2015-2016 Annual Report — Mark Morton
CTE’s 2015-2016 Annual Report is nearing completion and will soon be sent to the printers. It’s hard work creating the report, but also revealing and affirming: it gives us a chance to look back over the past year and discern what we have accomplished from a “big picture” perspective. And of course it also helps us reorient ourselves for a new year of activity.
As a preview of our 26-page report, I’ll paste below some of the achievements that our Director, Dr. Donna Ellis, highlights in her preamble to the report:
- Thanks to strategic plan funding, we hired a new Instructional Developer to assist with the development of our students’ communication skills. This staff member helps instructors at all levels learn strategies for teaching and assessing writing across the curriculum, as well as supports our instructional programs for graduate students.
- We contributed to two university-wide committees on large-scale change projects to assist with teaching quality: one on student evaluations of teaching and another on teaching and learning spaces. We bring research evidence and best practices to bear on these important and complex initiatives.
- In conjunction with the Graduate Studies Office, we launched a two-day Graduate Student Supervision series to ensure high-quality graduate instruction and assist new faculty members in attaining supervision status.
- With colleagues from Western University and Queen’s University, we developed two of six new online modules on university teaching for use in our instructional programs.
- We increased participation in our instructional development programming: since 2013, the number of unique participants in our workshops has increased by 19 per cent, with total workshop completions increasing by 37 per cent. This increase reflects an improved uptake, as our total number of workshops increased by only 23 per cent in the same timeframe.
- We added more instructor profiles to our high-traffic website to help promote public awareness of Waterloo’s teaching excellence.
- We started three projects to encourage innovative methods of course delivery using learning technologies. One project involves developing a new process for soliciting information from instructors about their use of learning technologies (beyond LEARN) so we can report on their usage and facilitate the sharing of best practices.
If you’re interested in receiving a copy of CTE’s 2015-2016 Annual Report, just let me know: mmorton@uwaterloo.ca .We’ll also be adding a link on our website to an accessible PDF version.
Artificial Teaching Assistants
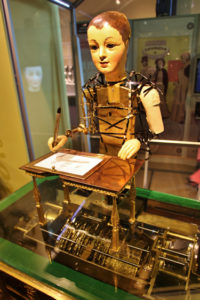
The dream of creating a device that can replicate human behaviour is longstanding: 2500 years ago, the ancient Greeks devised the story of Talos, a bronze automaton that protected the island of Crete from pirates; in the early thirteenth century, Al-Jazari designed and described human automata in his Book of Knowledge and Ingenious Mechanical Devices; in the eighteenth-century, the clockmaker Henri Maillardet invented a “mechanical lady” that wrote letters and sketched pictures; and in 2016, Ashok Goel, a computer science instructor at Georgia Tech, created a teaching assistant called Jill Watson who isn’t a human – she’s an algorithm.
Goel named his artificial teaching assistant after Watson, the computer program developed by IBM with an ability to answer questions that are posed in ordinary language. IBM’s Watson is best known for its 2011 victory over two former champions on the gameshow Jeopardy! In Goel’s computer science class, Watson’s job was to respond to questions that students asked in Piazza, an online discussion forum. Admittedly, the questions to which Watson responded were fairly routine:
Student: Should we be aiming for 1000 words or 2000 words? I know, it’s variable, but that is a big difference.
Jill Watson: There isn’t a word limit, but we will grade on both depth and succinctness. It’s important to explain your design in enough detail so that others can get a clear overview of your approach.
Goel’s students weren’t told until the end of the term that one of their online teaching assistants wasn’t human – nor did many of them suspect. Jill Watson’s responses were sufficiently helpful and “natural” that to most students she seemed as human as the other teaching assistants.
Over time – and quickly, no doubt – the ability of Jill Watson and other artificial interlocutors to answer more complex and nuanced questions will improve. But even if those abilities were to remain as they are, the potential impact of such computer programs on teaching and learning is significant. After all, in a typical course how much time is spent by teaching assistants or the instructor responding to the same routine questions (or slight variations of them) that are asked over and over? In Goel’s course, for example, he reports that his students typically post 10,000 questions per term – and he adds that Jill Watson, with just a few more tweaks, should be able to answer approximately 40% of them. That’s 4000 questions that the teaching assistants and instructor don’t have to answer. That frees up a lot of their time to provide more in-depth responses to the truly substantive questions about course content.
More time to give better answers: that sounds like a good thing. But there are also potential concerns.
It’s conceivable, for example, that using Watson might not result in better answers but in fewer jobs for teaching assistants. Universities are increasingly keen to save money, and if one Watson costs less than two or three teaching assistants, then choosing Watson would seem to be a sound financial decision. This reasoning has far broader implications than its impact on teaching assistants. According to a recent survey, 60% of the members of the British Science Association believe that within a decade, artificial intelligence will result in fewer jobs in a large number of workplace sectors, and 27% of them believe that the job losses will be significant.
Additionally, what impact might it have on students to know that they are being taught, in part, by a sophisticated chatbot – that is, by a computer program that has been designed to seem human? Maybe they won’t care: perhaps it’s not the source of an answer that matters to them, but its quality. And speaking for myself, I do love the convenience of using my iPhone to ask Siri what the population of Uzbekistan is – I don’t feel that doing so affects my sense of personal identity. On the other hand, I do find it a bit creepy when I phone a help desk and a ridiculously cheery, computerized voice insists on asking me a series of questions before connecting me to a human. If you don’t share this sense of unease, then see how you feel after watching 15 seconds of this video, featuring an even creepier encounter with artificial intelligence.
Communities of Practice — Rudy Peariso (Centre for Extended Learning)
Community! Not often the first word that comes to mind when thinking of online learning, but it is for a group of like-minded instructors at the University of Waterloo. The inaugural meeting of the Online Instructors Community of Practice took place during the last week of April.
Sometimes online classes can have the reputation of being solitary for both teachers and learners. Although at the Centre for Extended Learning we work with instructors to dispel that myth for learners, we hadn’t fully considered the impact that online teaching has on instructors. One of our instructors was looking for advanced workshops and a way to share her experiences, and the Online Instructor Community of Practice was born.
Wenger, McDermott, and Snyder (2002) define a Community of Practice as “groups of people who share a concern, a set of problems, or a passion about a topic, and who deepen their knowledge and expertise in this area by interacting on an on-going basis.”
Over lunch, hosted by the Centre for Extended Learning (CEL), nineteen instructors, who teach online at the University of Waterloo, discussed the successes and challenges of teaching online. Topics on student engagement, teaching presence, academic integrity, and blended learning all emerged. Community members were overheard talking about how nice it was just to talk to others who had the same challenges and successes as they experience.
CEL is actively looking at ways to enhance the community, and have opted to offer a meeting once per term. Suggestions for the meeting include, a show and tell, select topics and a discussion of dilemmas. A newly created listserv gives instructors the opportunity to share suggestions and ask questions of the community.
If you currently teach online and want to join the Community of Practice, contact the Centre of Extended Learning.
If you are interested in establishing a Community of Practice for your discipline or interest, check out the following resources:
- What is a Community of Practice?
- Wenger, E. (2014) Communities of practice: a brief introduction
- Wenger, E. C., McDermott, R., and Snyder, W. C. (2002). Cultivating Communities of Practice: A Guide to Managing Knowledge. Harvard Business School Press: Cambridge.
Image by Niall Kennedy, Creative Commons License.
What We Can Only Learn from Others — Donna Ellis, CTE Director
You know when you have an “a-ha” moment and two ideas from completely different contexts suddenly merge in your mind? I had this happen to me when I attended a recent faculty panel discussion in Math about the use of clickers. The panelists shared a variety of experiences and gave excellent advice to their colleagues. My “a-ha” moment arose when the panel facilitator declared how much she had learned about her students when she started to use clickers: “I thought I knew what they were thinking. Boy, was I wrong!” Her statement cemented for me the extreme value of asking others about their thinking rather than making assumptions and then devising plans based on those assumptions.
You may have heard that CTE is going to have an external review in 2017. It’s time and it’s part of our institutional strategic plan for outstanding academic programming. Our Centre was launched in 2007, a merger of three existing units that supported teaching excellence. Many things have changed since then, including the structure of our leadership, our staffing, the breadth of services that we provide, and our location. Organic, evolutionary change is positive, but there’s value in stepping back to see where we’ve been, what’s on the horizon, and how to get there. And this is where the “a-ha” moment comes in: my small CTE team working on this review cannot know what others think about where we are and where we could go. I’ve always known this, but it’s one thing to know it and another to do something about it.
And so we’ll be asking, both as we prepare for our self-study and during the external reviewers’ visit. We have already started to ask some different questions on our feedback instruments about our services, focusing on ways that working with us have helped to enhance your capacity and your community as teachers. These changes are part of launching a comprehensive assessment plan that connects to our Centre’s overall aims. But we have also begun to work on sets of questions for our external review about areas that we might be too close to see clearly or cannot know because the responses needed are others’ perceptions. These questions involve topics ranging from our mission statement and organizational structure to our relationships with others and the quality of our work. We also need input on the possibilities for “CTE 2.0”: where could we be in another 10 years?
We’ll be starting this data collection with our own staff members, doing a SWOT analysis (strengths, weaknesses, opportunities, and threats) this spring term. But we will be seeking input far beyond our own walls, including beyond UWaterloo. When we come knocking (literally or by email or by online survey), I trust you’ll answer and provide your honest feedback and insights. We believe we are a responsive organization that helps those who work with us to achieve their goals, and we have some data to support these claims, but we want more. We want your input. We want to be able to say: “We didn’t know that. We’re so glad we asked!”
If you have thoughts or insights into our external review plans, please let me know. You can reach me at donnae@uwaterloo.ca or at extension 35713. We want to make this external review activity as generative and useful as possible. I am optimistic that with your help we can achieve just that.
Photo courtesy of David McKelvey
High Failure Rates in Introductory Computer Science Courses: Assessing the Learning Edge Momentum Hypothesis – John Doucette, CUT student
Introductory computer science is hard. It’s not a course most students would take as a light elective, and failure rates are high (two large studies put the average at around 35% of students failing). Yet, at the same time, introductory computer science is apparently quite easy. At many institutions, the most common passing grade is an A. For instructors, this is a troubling state of affairs, which manifests as a bimodal grade distribution — a plot of students’ grades forms a valley rather than the usual peak of a normal distribution.
For most of the last forty years, the dominant hypothesis has been the existence of some hidden factor separating those who can learn to program computers from those who cannot. Recently this large body of work has become known as the “Programmer Gene” hypothesis, although most of the studies do not focus on actual genetic or natural advantages, so much as on demographics, prior education levels, standardized test scores, or past programming experience. Surprisingly, despite dozens of studies taking place over more than forty years, some involving simultaneous consideration of thirty or forty factors, no conclusive predictor of programming aptitude has been found, and the most prominent recent paper advancing such a test was ultimately retracted.
The failure of the “Programmer Gene” hypothesis to produce a working description of why students fail has led to the development of other explanations. One recently proposed approach is the Learning Edge Momentum (LEM) hypothesis, by Robins (2010). Robins proposes that the reason no programmer gene can be found is because the populations are identical, or nearly so. Instead of attributing the problem to the students, Robins argues that it is the content of the course that causes bimodal grade distributions to emerge, and that the content of introductory computer science classes is especially prone to such problems.
At the core of the LEM hypothesis is the idea that courses are composed of units of content, which are presented to students one after another in sequence. In some disciplines, content is only loosely related, and students who fail to learn one module can still easily understand subsequent topics. For example, a student taking an introductory history class will not have much more difficulty learning about Napoleon after failing to learn about Charlemagne. The topics are similar, but are not dependent. All topics lie close to the edge of student’s prior knowledge. In other disciplines however, early topics within a course are practically prerequisites for later topics, and the course rapidly moves away from the edges of students’ knowledge, into areas that are wholly foreign to them. The more early topics students master, the easier the later ones become. Conversely, the more early topics that students fail to acquire, the harder it is to learn later topics at all. This effect is dubbed “momentum.”
Robins argues that introductory computer science is an especially momentum-heavy area. A student who fails to learn conditionals will probably be unable to learn recursion or loops. A student who fails to grasp core concepts like functions or the idea of a program state will likely struggle for the entire course. Robins argues that success on early topics within the needed time period (before the course moves on) is largely random, and shows via simulation that, even if students all start with identical aptitude for a subject, if the momentum effect is increased enough, bimodal grade distributions will follow. However, no empirical validation of the hypothesis was provided, and no subsequent attempts at validation have been able to confirm this model. The main difficulty faced in evaluating the LEM hypothesis is that the predictions it makes are actually very similar to the “Programmer Gene” hypothesis. Both theories predict that students who do well early in a course will do well later on. The difference is the LEM hypothesis says this was mostly down to chance, while the “Programmer Gene” hypothesis says it was due to the students’ skill.
In my research project for the Certificate in University Teaching (CUT), I proposed a new method of evaluating the LEM hypothesis by examining the performance of remedial students — students who retake introductory computer science classes after failing them. The LEM hypothesis predicts that remedial classes should also have bimodal grade distributions, because student success on initial topics is largely random. Students taking the course for the second time should be just as likely to learn them as students taking the course the first time round. In contrast, the “Programmer Gene” hypothesis predicts that remedial courses should have normally distributed grades, with a low mean. This is because remedial students lack the supposed “gene”, and so will not be able to learn topics much more effectively the second time than they were the first time.
To evaluate this hypothesis, I acquired anonymized data from four offerings of an introductory computer science course: two with a high proportion of remedial students, and two with a very low proportion. I found weak evidence in support of the LEM hypothesis, as all grade distributions were bimodal when withdrawing students were counted as failing. However, when withdrawing students were removed entirely, only one non-remedial offering was bimodal, a result predicted by neither theory.
Although my empirical results were ultimately inconclusive, my research provides a clear way forward in evaluating different hypotheses for high failure rates in introductory computer science. A follow up study, conducted with data from a university that offers only remedial sections in the spring term (removing the confounding effects of out-of-stream students in the same class) may be able to put the question to rest for good, and facilitate the design of future curricula.
References:
Robins, A. (2010). Learning edge momentum: A new account of outcomes in CS1. Computer Science Education, 20 (1), 37-71.
The author of this blog post, John Doucette, recently completed CTE’s Certificate in University Teaching (CUT) program. He is currently a Doctoral Candidate in the Cheriton School of Computer Science.
New Tool for Making Screencast: MyBrainShark
Screencasts are an educational technology that have accelerated from zero to sixty in a relatively short time — in fact, over just the past few years. Screencasts have the potential, too, to radically change education. For one thing, they are the technology behind the pedagogical notion of “flipping” the classroom — that is, of providing content to students outside of class via screencasts, and reserving class time for more engaging activities that leverage application of knowledge, peer instruction, and collaboration. The word “flipping” almost sounds glib, but the pedagogical change it embodies is revolutionary: it threatens to upend what higher education has been for the past, oh, thousand years.
In my workshops on screencasts, I usually refer to Camtasia, Adobe Presenter, and Screencast-O-Matic as good tools for creating screencasts. Camtasia is a good choice at the University of Waterloo because we have a site license for it, so you can buy an inexpensive copy at The Chip. Screencast-O-Matic is a viable option for those who want to test the waters: its fully online (nothing to download) and free; it has limited editing capabilities, but it will give you a sense of what you might do with screencasts in your courses.
Just a few weeks ago, I also discovered another screencasting tool that I would recommend: MyBrainShark. This tool is perfect if you already have a PowerPoint presentation and want to record narration for it. It’s free, fully online, and dead simple to use. I also like the fact that links that are embedded into the PPT presentation remain “live” after the presentation has been converted into a MyBrainShark screencast.
You can see an example of a MyBrainShark screencast here (it’s a screencast about “glimpse concepts” and their relevance to smart phones).